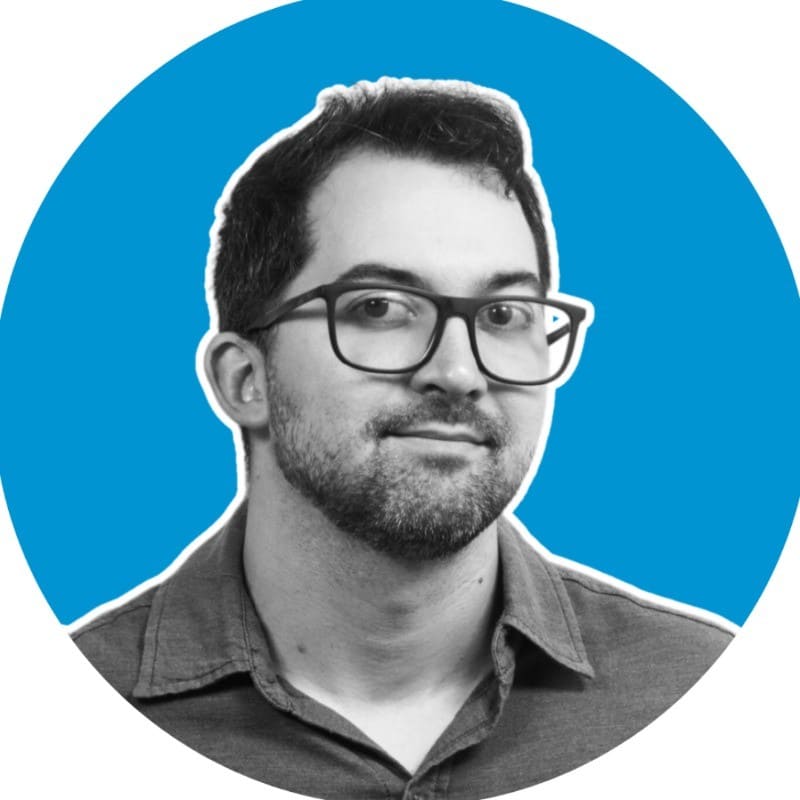
By
Raphael Santos
January 31, 2023
Updated
September 12, 2023
Source: Frame Stock Footage/Shutterstock
Structuring a machine learning team with the right roles is key to a successful long-term tech strategy
Data science and artificial intelligence are integral components of many businesses and the demand for machine learning teams has been rising. To truly leverage the benefits that come from incorporating artificial intelligence and machine learning (ML) into their operations and products, organisations need to build an effective ML team.
Creating a successful machine learning team requires thoughtful consideration when it comes to staffing and skill requirements. In this article, we’ll take an in-depth look at the different roles within an ML team, as well as provide advice on building a strong one.
1. First steps for successful machine learning team building
1.1 Begin by defining your machine learning project goals
1.2 Next, define the type of model needed to solve your business challenges
1.2.1 These are the characteristics of an effective model
2. Decide how you'll build your machine learning team
4. Monitor your market transformation closely
5. Identify the right roles to fill
5.1 This is how a strong teamlooks like
5.2 Product Manager: don't underestimate the value of leadership
6. Develop strategies for hiring qualified staff
6.1 We can help you hire a top talented workforce
First steps for successful machine learning team building
It's important to be aware of your company's objectives and operations before onboarding any new talent. Having a clear vision of the challenge ahead enables you to form the right team to tackle it.
Begin by defining your machine learning project goals
For a machine learning project to be successful, you need to identify the business problem you're attempting to solve and gather the data which will be used to train a model — a mathematical representation of a system or process.
Source: Frame Stock Footage/Shutterstock
The model is used to make predictions and decisions based on data. In machine learning, models are trained using data sets to learn patterns and relationships between different variables.
In order to meet your business needs, it's important to be knowledgeable about the various models that are available.
Next, define the type of model needed to solve your business challenges
Once you have a clear goal for your project, you can start to think about the types of models that will be used. Different models are suited to different tasks, so it’s important to understand what each model can do and how it can be used in your project.
View our chart below to see how you can utilise different models (e.g. automation, annotation, extraction) in a larger system.
Most business challenges that can be addressed with machine learning can be put into one of the forms from the list. If your problem does not fit any of these, ML may not be the ideal solution.
These are the characteristics of an effective model
A successful model must meet specific input and output requirements as well as be efficient. It must also help the enterprise by lowering costs, increasing sales or profits, enhancing user productivity engagement and satisfaction, and of course, it must be scientifically vigorous.
To gain insight into scientific rigour, a calculator can serve as a useful analogy. Inputting the same numbers over and over again should give us the same result each time, demonstrating dependability and reproducibility.
Even though the problem appears straightforward, solving it can be complex, especially when you're working with binary models. Establishing a category could be problematic, as the model requires the capability to separate between two types, so including the class "Other" could be of assistance.
Moreover, achieving a unified objective is an arduous task. Each segment of your business will possess divergent objectives from the project. The finance team may want something distinct (e.g. fraud detection, risk management and etc.), whereas the marketing unit some other thing (e.g. segment targeting, life time value forecasting and etc.) and so on.
Consequently, finding a fine equilibrium between these goals and translating the data is a real challenge.
Decide how you'll build your machine learning team
Once you’ve identified the goals you want to achieve and the model best suited for them, it's easier to figure out what type of machine learning team is needed.
Depending on the organisation, two methods for forming a machine learning team exist.
One approach involves data analysts who work collectively with software developers. In this system, a software engineer does not need in-depth knowledge on machine learning; however, they must be familiar with the language of other data professionals or researchers.
The other method calls for every engineer on a machine learning squad to have proficiency in both machine learning and software engineering.
The advantages and disadvantages of both options are evident. The first favours an approach that allows each team member to specialise in a particular area, while the second believes that combining abilities produces better project outcomes.
In either case, it is important to have a team that is able to communicate effectively and work together. This includes having a workplace strengthened by the development of soft skills.
Cultivate soft skills
By developing and cultivating soft skills such as communication and collaboration, a machine learning team can perform much better.
Source: Frame Stock Footage/Shutterstock
It helps to refine the storytelling aspect of data so that stakeholders can better understand the project. Soft skills also help to translate technical concepts into clear visuals for executive decision-makers.
Last but not least, it helps to foster greater communication within the team among the members by creating an environment of trust and openness. With everyone on the same page exploring projects together, the work process become more efficient and enjoyable.
Monitor your market transformation closely
Additionally, it is important to stay knowledgeable about the business environment and the latest staffing trends. For instance, there is a current migration towards developing green technology solutions, which has created a competitive landscape for energy transition-related roles that can be decisive for businesses.
In light of this conversation, we recently created a whitepaper to help companies stay informed about the popular skills and the talent needed in green tech. You can download the whitepaper, "Green Tech Talent Required to Boost Net Zero", for free below.
Identifying the right roles to fill
Identifying these roles require an understanding of what skills are most critical for the successful deployment of machine learning solutions. There should be individuals with diverse backgrounds that can bring together technical knowledge and domain expertise.
This is how a strong squad looks like
A strong machine learning squad need to be complementary. It has to possess roles that can supplement each other. So, if you're preparing to create many powerful machine learning projects, these are the roles you can look to fill to keep up with the current trends:
- Machine Learning Engineer
- Data Scientist
- Data Engineer
- Product Manager
- Machine Learning Researcher
- DevOps Engineer
- MLOps Engineer
This team structure is very robust and yet, only a few workplaces today have such a complete squad. The tight job market and the expensive salaries in technology can lead to companies having difficulty in finding qualified ML professionals.
Nevertheless, this entire structure will help you determine what your budget can afford while also meeting your current business needs with the right roles.
Product Manager: don't underestimate the value of leadership
A strong machine learning team is like an orchestra. It needs both the conductor and the musicians to come together in harmony to present a beautiful performance. And you've guessed correctly to point the Product Manager as the maestro behind this.
Great leadership is essential in any team, especially when it comes to machine learning. A lack of creative vision and clear direction can be extremely detrimental to a project’s success and objectives. The Product Manager sets the tone: clarifying goals, managing expectations, and inspiring collaboration between team members.
They must have the ability to stay organised amidst chaos and think strategically by planning out a course of action that makes sense for the entire team. In order for an ML project to succeed, having the right leadership is just as important as having great developers and data scientists on the stage.
Develop strategies for hiring qualified staff
When building a strong machine learning team, it is important to have the right mix of skills and expertise. To ensure that the team has the necessary qualifications, it is important to develop strategies for hiring qualified staff.
Partnering with an Employer of Record is a great way to broaden your recruitment horizons. Through their Global Employment Services, these firms provide access to a global talent pool and are able to support businesses throughout the entire relocation process. They can also assist with ensuring compliance in terms of taxes, benefits and labour laws.
This means finding staff who have the right skill sets and qualifications and getting them aboard quickly and easily — providing a competitive advantage to any organisation no matter its location.
We can help you hire a top talented workforce
At Airswift, we specialise in providing world-class recruitment solutions for the engineering and technology sectors. With more than 60 offices and over 9,000 contractors worldwide, we are the ideal partner to help you achieve your goals from expanding your workforce to broadening your business.
Building a strong machine learning team is a time-consuming task made all the more difficult by the technology talent crunch. For this reason, it is advantageous to engage an experienced recruiting partner to help overcome these obstacles.